How the MDRC Center for Data Insights Is Improving Programs and Systems with Actionable Evidence
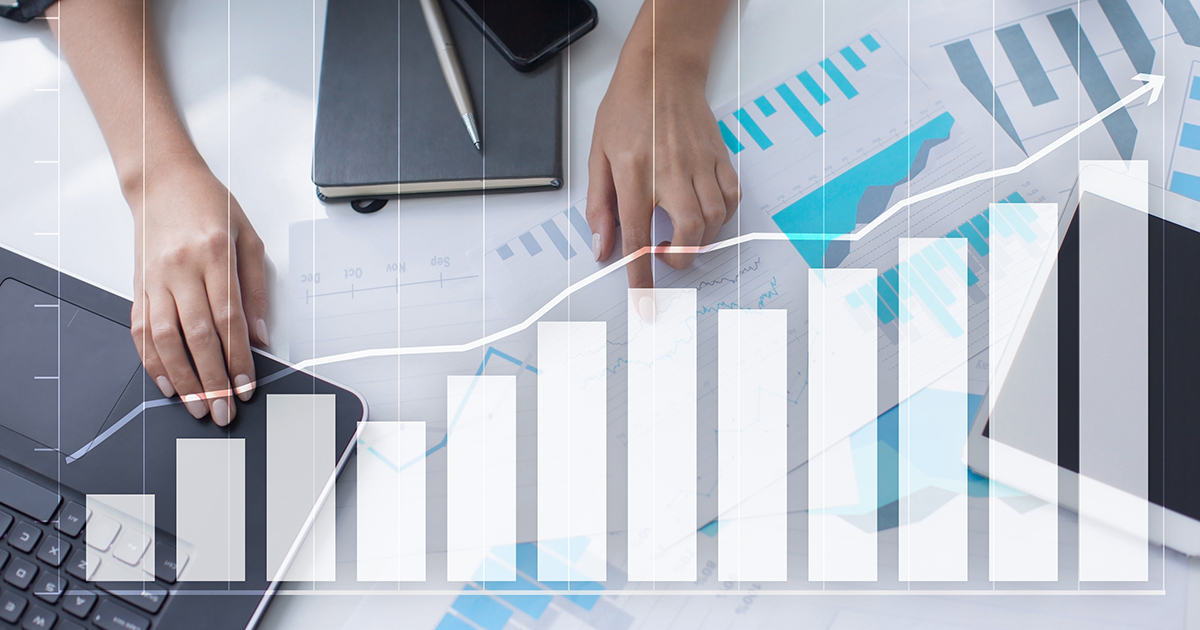
MDRC has more than four decades of experience in developing, evaluating, and improving social and education programs, as well as in managing and analyzing vast amounts of data in ways that meet the highest standards of responsibility, security, and privacy. With the creation of the MDRC Center for Data Insights, MDRC is furthering its long-standing commitment to helping our partners improve their programs and systems. Ranging from simple descriptive summaries to advanced machine learning algorithms, the Center’s eight current projects aim to use institutions’ increasingly rich data to provide new insights that can help them refine and target their services:
Temporary Assistance for Needy Families Data Innovations (TDI) is supporting innovation and effectiveness of state-level Temporary Assistance for Needy Families (TANF) programs by enhancing the use of data from TANF and related human services programs. This work will include strengthening state integrated data systems, promoting proper payments and program integrity, and enabling data analytics for TANF program improvement. Across its activities, the project will support the use of data for understanding the broad impact that TANF has on families and will improve knowledge of how the federal government and state partners can use data to more efficiently and effectively serve TANF clients. Partners include Chapin Hall at the University of Chicago, the Center for Urban Science and Progress at New York University, and Actionable Intelligence for Social Policy at the University of Pennsylvania. Launched in 2017, TDI is sponsored by the Office of Planning, Research, and Evaluation in collaboration with the Office of Family Assistance in the Administration for Children and Families, U.S. Department of Health and Human Services.
Partnership with the Center for Employment Opportunities (CEO) for Predictive Analytics is harnessing granular, longitudinal administrative data to build a system for ongoing, advanced analytics that support CEO’s continuous improvement process. Foremost, the project is using predictive analytics to provide early warnings to front-line workers of participants’ risks of not reaching milestones in CEO’s employment training program for former prisoners reentering society. CEO plans to train staff members to act on this information and to work with MDRC to design, implement, and test new interventions based on insights provided by the predictive analytics results. The project is also incorporating additional, iterative, and automated data analytics that will provide real-time monitoring of program outcomes. These analytics capabilities include attendance and attrition reports, A/B testing, and data visualization.
Subprime Lending Data Exploration Project, a “big data” project funded by the MetLife foundation, is designed to produce policy-relevant insights using an administrative data set that covers nearly 50 million individuals who have applied for or used subprime credit. The data set contains information on borrower demographics, loan types and terms, account types and balances, and repayment histories. To investigate whether there were distinct groups of borrowers in terms of loan usage patterns and outcomes, MDRC used a data discovery process called K-means clustering. The project used several other techniques to derive insights into payday lending behavior, including geospatial analysis and conjoint experiments. More recently, the project has exploited the enormous scale of the database to analyze cross-border variation in payday lending usage as a function of whether states took advantage of the Medicaid expansion.
The Pretrial Justice Reform Study is assessing and potentially improving a pretrial assessment tool developed by the Laura and John Arnold Foundation that predicts defendants’ risks of failure to appear for court, committing a new crime while awaiting court, and committing a new violent crime. The tool aims to help judges make more informed, less subjective decisions about pretrial detention. It is currently used in nearly 40 jurisdictions across the nation. The project team brings a unique combination of substantive and methodological expertise in cutting-edge predictive analytics methods, while also understanding the pretrial criminal justice context and the practical considerations that could affect the usefulness of a risk tool in various jurisdictional contexts and its potential to expand to a larger scale.
New Visions for Public Schools (NVPS) Researcher-Practitioner Partnership for Predictive Analytics is a partnership in which MDRC researchers developed and implemented a comprehensive predictive modeling framework that allows for rapid and iterative estimation of a continuous measure of risk for each student at a point in time. The framework was implemented with data from NVPS’s network of 70 high schools to estimate students’ risk of not graduating on time and of not passing the state algebra exam required for graduation.
Using Machine Learning to Predict Third-Grade Reading Proficiency is a project with the Wake County Public School System. MDRC researchers are applying our machine learning approach to predicting third-grade reading proficiency, using DIBELS reading tests and subtests. Our preliminary investigation suggests that predictive analytics with machine learning can do what humans cannot — combine and weight many pieces of information in a way that is optimal for making predictions. Applying these methods allows educators to more accurately detect at-risk students who can benefit from intervention — without generating substantial false positives. The next steps will be to examine whether the accuracy of these models is improved by adding other information about these students, such as measures of their attendance and tardiness and other data schools collect internally or integrate from other sources.
The Long-Term Outcomes Project is an effort to produce new findings from older studies using new matching techniques and approaches. The project is assessing the feasibility of linking administrative data sets to program evaluation records, a promising and potentially low-cost means of tracking the long-term impacts of social interventions. While social programs are often designed to have long-term benefits for participants, many evaluations do not (or are not able to) track outcomes in the long term. With recent interest in making administrative data more accessible — reflected in the recommendations of the Commission on Evidence-Based Policymaking — it is important for researchers and others to understand whether and how these data sets can be linked to evaluation data sets. For the Long-Term Outcomes Project, data are being collected from 16 employment-related evaluations to assess the practical and legal feasibility of accomplishing these links, to assess potential costs, to determine who owns various sources of data, to identify any history of links to other projects, to catalogue past findings, and to gauge the current availability of relevant data and metadata.
Chicago Community Networks Study, funded by the MacArthur Foundation, uses social network analysis to explore ways to consider power in networks of neighborhood organizations, how power is configured differently in different Chicago neighborhoods, and how these patterns can help communities respond to local challenges. Social network analysis can allow researchers to understand how neighborhoods differ in the levels and extents of these interactions across domains — how “comprehensive” community connections really are. MDRC has produced state-of-the-art network graphics to help measure comprehensiveness in ties among local organizations in Chicago neighborhoods, and to show how comprehensiveness can help neighborhoods work together to build needed affordable housing and improve schools.