Research Transparency and Replication at MDRC
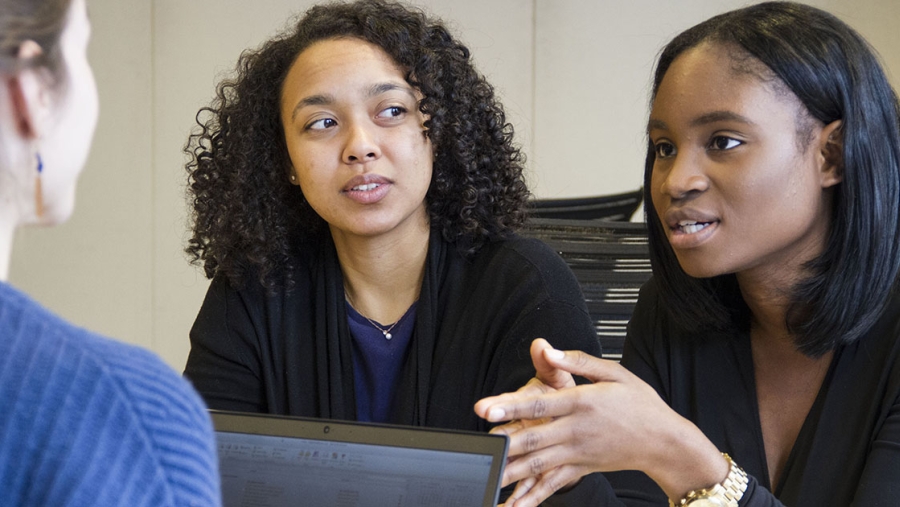
This post is one in a series highlighting MDRC’s methodological work. Contributors discuss the refinement and practical use of research methods being employed across our organization.
Many researchers are concerned about a crisis in the credibility of social science research because of insufficient replicability and transparency in randomized controlled trials and in other kinds of studies. In this post we discuss some of the ways that MDRC strives to address these issues and ensure the rigor of its work.
An important tenet of building reliable evidence about social policy and programs (and of science in general) is that research findings can be both reproduced and replicated and that the methods and data that produce findings are transparent. Reproducibility refers to the ability of other researchers to produce the same results with the same data. Replicability refers to the ability to repeat a study and obtain the same results, or at least results of similar magnitude for the same outcomes, with new data. Both are important to ensure that policymakers, practitioners, and the public can have confidence in the evidence. Transparency allows other researchers to assess the methods, reproduce and replicate the studies, and advance knowledge by generating and testing new hypotheses.
In the last several years, the results of some studies have come into question because they could not be either replicated or reproduced, for a variety of reasons. For example, in a test of 100 peer-reviewed psychology studies from three top journals, only 39 replications by different researchers met the criteria for success; in a political science paper about voter persuasion, a set of researchers found faked data; and an influential economics paper was found to have data errors that led to incorrect results. Problems of reproducibility — as in the economics paper — are often due to data errors; replication problems also may be due to errors or to poor research design and practice, or they may indicate that the findings were applicable only to a specific sample at a specific time. Even if a study is conducted ethically and without error, if it took place in a unique setting or with a unique population, the findings may not be generalizable. The ability to repeat the findings in other environments increases the robustness — and usefulness — of the results.
In response to these issues of credibility and generalizability, the social science research community is moving to adopt practices that address various facets of the problem. Given our mission to produce reliable evidence in service of social policy, MDRC embraces this effort and follows several practices in the design of our randomized controlled trials and strong quasi-experimental studies:
Prespecifying and preregistering research plans. At MDRC, it is standard practice to prespecify analysis, writing down all the steps we plan to implement for data cleaning and analysis before doing either. (Ideally this process happens even before we begin collecting data, but sometimes that is not feasible.) We have recently begun using a company-wide template for this purpose, which requires designating primary outcomes and subgroups, defining measures and transformations, establishing rules for sample creation and for addressing missing data, and specifying parameters of interest, estimation models and procedures, and hypothesis tests.
Prespecifying obliges and permits researchers to (1) elucidate the goals of their study, (2) support the scientific integrity of their findings by minimizing the possibility of “fishing” for positive findings via multiple specifications, (3) allow others to assess the validity of their methods, (4) circumvent publication bias against studies without significant findings, and (5) allow for reproducibility and replication. Some funders and some journals now require analysis plans to be registered publicly at a site such as the American Economic Association's registry for randomized controlled trials, the Society of Research on Educational Effectiveness registry, the Open Science Framework, the federal government’s ClinicalTrials.gov, or the Evidence in Governance and Politics registry.
Requiring more than statistical significance. Findings in experimental studies are typically designated as effects, or impacts, when the p-values for corresponding hypothesis tests are smaller than the prespecified threshold of statistical significance (usually 0.05 or 0.10). A p-value of 0.10 means that there is only a 10 percent chance that a program with no effect would have produced such a large estimate. But small changes in the steps of the analysis (such as sample restrictions or model specification) can change the p-value. To reduce the risk of drawing incorrect conclusions based on a false positive, we have several practices to guide our interpretation of findings based on all the evidence:
-
We use a short list of outcomes and subgroups in our primary analyses to protect against spurious findings due to multiple hypothesis testing, where the number of impact estimates examined (multiple outcomes or subgroups) increases the likelihood of at least one false positive.
-
When we do examine multiple outcomes, we use statistical adjustments to control the rate of false positives.
-
We perform sensitivity tests to investigate whether different assumptions call the findings into question.
-
In addition to the point estimate, we look at the confidence interval around the estimate, which indicates its precision.
-
We report most, if not all, of our estimates as effect sizes, calculated in terms of the standard deviation of the outcome, which helps us understand the substantive significance of a finding.
-
We consider whether we have similar results for similar outcomes: If an estimate for just one outcome is significant, and estimates for related outcomes lack sufficient evidence of an effect, there is cause for skepticism.
-
We conduct most of our impact studies in conjunction with implementation research to understand the factors that may drive impact results, whether they are positive, negative, or null.
Sharing data. At MDRC, we frequently produce public use files from our data, allowing other researchers to reproduce our analyses and findings, conduct robustness checks with other methods, or explore additional hypotheses. We sometimes also share these files with interested practitioners (such as a school district where a study was conducted) who may want to use the data they helped us collect to further understand their own programming.
Conducting replication studies. We have also begun undertaking replications of some of our most well-known studies. For example, MDRC’s landmark Career Academies study is often cited in the literature on career and technical education. That study, which began in the early 1990s and followed students for eight years after high school graduation, found sustained impacts on earnings for students who had been randomly assigned to enroll in a career academy in high school. Now MDRC is replicating that research with our Next Generation California Partnership Academies study. The research team is currently working with schools to randomly assign students to participate in academies in California. The study will follow students through high school and into the workforce, with final results anticipated in 2030. This study will help us understand whether the Career Academy model continues to be successful for students, given the changes in the educational and workforce landscapes in the two decades since the original study participants enrolled.
In addition, MDRC conducts many large projects that study the same policy or program in multiple settings simultaneously. We strive to build a portfolio of studies on a topic: for example, the sequence of state welfare-to-work studies done in the 1980s and early 1990s, which showed a consistent pattern of findings. The more that findings are repeated, the more confidence we have in the results.