THE-RCT Database: A New Resource for Analyzing Studies of Postsecondary Education Interventions
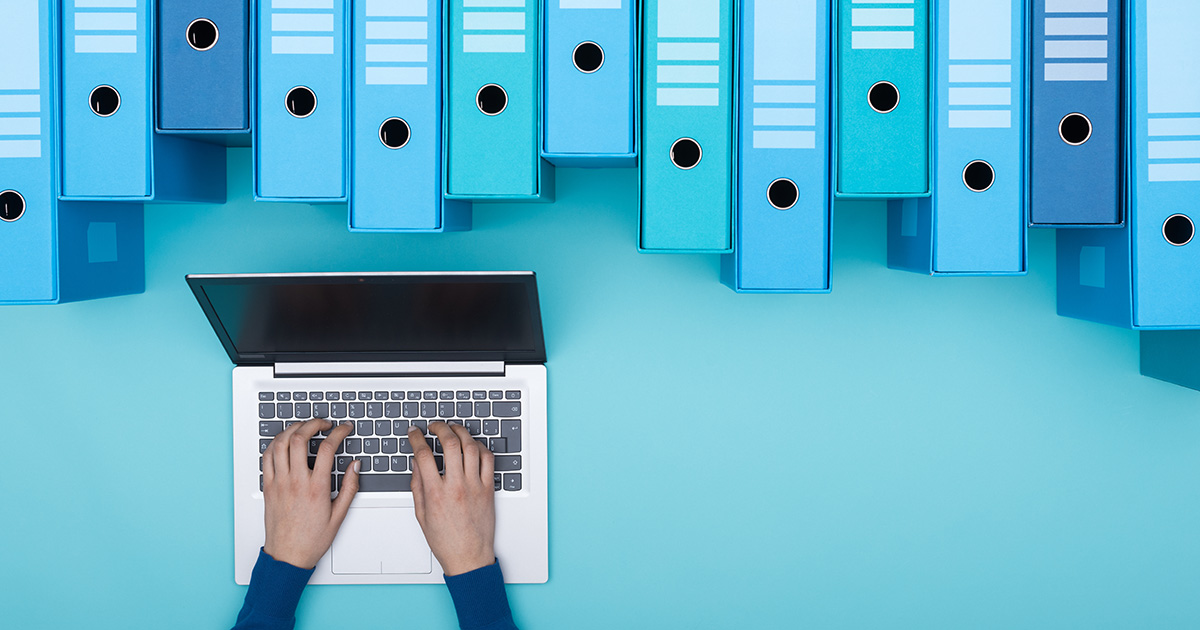
Improving outcomes for community college students has long been the focus of rigorous research studies by MDRC and others. Through a project called The Higher Education Randomized Controlled Trial, or THE-RCT, MDRC has created a broadly accessible database that compiles student-level data from all MDRC’s randomized controlled trial evaluations of postsecondary education programs. Researchers are able to use the database to conduct analyses across studies to answer important questions about the effectiveness of different higher education interventions. THE-RCT is supported by Arnold Ventures and the Institute of Education Sciences at the U.S. Department of Education.
In this episode, Leigh Parise talks with Michael Weiss, a Senior Fellow in MDRC's Postsecondary Education policy area, about how MDRC has used this database, how other researchers can access it, and how MDRC is encouraging colleagues to contribute their own studies to THE-RCT.
View Transcript
Leigh Parise: Policymakers talk about solutions, but which ones really work? Welcome to Evidence First, a podcast from MDRC that explores the best evidence available on what works to improve the lives of people in poverty. I'm your host, Leigh Parise.
Postsecondary education offers an important pathway out of poverty and into better jobs, but a host of factors, including inadequate financial aid or student services, can keep students from enrolling in and graduating from college.
Over the past two decades, MDRC and other researchers have conducted numerous rigorous evaluations to learn what works to improve outcomes for college students. Through a project called The Higher Education Randomized Controlled Trial, or THE-RCT, MDRC has created a broadly accessible database that compiles data from all of MDRC's evaluations. Researchers are able to conduct analyses across studies and answer important questions about the effectiveness of different higher education interventions, such as which program features seem to make the most difference for student success.
To learn more about the project I spoke with Mike Weiss, a senior fellow in MDRC's postsecondary education policy area. Hi Mike, thanks for joining me today. It's really great to have you.
Mike Weiss: Thanks, Leigh. It's great to be here with you.
Leigh: MDRC recently launched The Higher Education Randomized Controlled Trial project, also known as THE-RCT. I wonder which clever person came up with that name, listeners. It was Mike. Mike, tell me about the project.
Mike: Yeah, thanks. I did come up with a name, and I can't decide if I'm either ashamed about that or if it's actually great. But the project, THE-RCT as you said, started because around 20 years ago, we ran what we believed to be the first large scale randomized controlled trial in postsecondary education. There were around 1,500 students in a trial that took place at Kingsborough Community College here in New York City.
And we think that was a really important shift in the way that people look at evidence in postsecondary education. Since that time, there's been a lot more randomized controlled trials that are used to try to better understand the effectiveness of different programs, policies, practices in this space. Since that time, the last 20 years, we've conducted around 31 randomized controlled trials in postsecondary education, of 39 different interventions, including over 65,000 students across 45-plus institutions.
And we think that that information is really valuable in and of itself. And we've produced a lot of papers and reports on it. But this initiative was to put all that data together in one place, so that we could do some synthesis and look across all those studies, but also that other people could do it as well. So, we think it's a really exciting initiative. It's now the largest database of its type in postsecondary education. And we're hopeful that a lot of new learnings will come out of this that can help improve policies and practices that affect low-income Americans.
Leigh: I want to ask a really specific question. I know that this project is really focused on studies that have been randomized controlled trials or RCTs—why those specifically?
Mike: MDRC, in general, one of our specialties is conducting randomized controlled trials. And in the postsecondary space, we've done, I think, more of them than any other individual and/or firm or college in the country. So that's one reason why we just have access to a lot of these.
But the real thing is that: this is a really great research method to try to learn about the effectiveness, the causal effectiveness that this intervention actually causes different changes and outcomes. It's a really great approach to kind of answer those questions about whether or not a program or policy is working, whether or not it's making a difference, whether or not it's making people's outcomes be different than they would have been had they not experienced a particular program policy intervention. So it's a very powerful tool to try to learn about the effectiveness of interventions.
And putting all these studies together into a big database, where we've done a really good job, I think, about learning causally, whether or not those interventions, what effects they were having, we hope will help improve the research that others can do looking across those studies.
I'll also note the federal government that funds the Institute for Educational Sciences and the What Works Clearinghouse, they have certain standards that they believe kind of provide the best level of evidence about the effectiveness of different programs. And they are a big fans of randomized controlled trials when you're trying to understand the effectiveness of interventions.
And one kind of nice thing about these studies and this database, is that this What Works Clearinghouse that says, "You've kind of met our seal of approval." They have found that I want to say like 20 or so of the interventions in our database, they meet their standards without reservations. The ones that they haven't said that about, it's actually not because they don't meet their standards, they just haven't reviewed those studies yet.
And given the design and the way all these studies have worked out, my guess is every single one of them meets this highest level of standards set forth by the federal government in terms of evidence standards. So these really are, I think, some of the highest-quality research studies that have been done in postsecondary education about the effectiveness of different programs, policies, practices.
Leigh: It's nice that anybody using this database knows for sure that one thing in common across all the evaluations is that they all use this lottery-like process to create equal groups, so that we can be sure that whatever intervention or interventions are being tested are actually what caused any difference in outcomes that we see. Mike, can you say a little bit more about why there's a need for this kind of database?
Mike: There's a few reasons. One of the main reasons is that it enables us to look beyond just any individual study. So oftentimes when we're working on a study, we're trying to find out: does program X make a difference? Is it helping students earn more credits, continuing college, graduate? But we sort of sometimes forget to look more at the bigger picture, look across these studies to synthesize what we're learning.
And putting all this data together in one place enables both us and other researchers to do that, to look across studies and see if there's more that can be learned than what you learn from any one individual study.
This kind of thing comes up a lot. There's the current replication crisis where oftentimes you do an evaluation of a program, the results look fairly positive. And then it's not until you try it again at more and more places that you learn a little more about…perhaps that first study was a fluke is one thing that could happen, or perhaps it wasn't a fluke, but it's that the program did work in one context, but not in another. Or if it was implemented differently in some other context, maybe it works in one and then not in the other and so forth. So, there's many different reasons that we can gain some substantive knowledge by looking across more than just one study.
In addition, though, there's also a big open science movement that's going on. And I think that they're in some ways related to that point about replication—but there's been a big push towards making more data available so that other researchers can access it, make use of it, and learn more from it.
Some of this can be [that], even though we do the best job we can in our studies of analyzing the data and interpreting it as well as we can, others might look at it differently. And I think that can actually benefit the scientific community and what we learn from these studies— so, enabling other researchers to check our work or look at it from different angles, we think could be really valuable.
And then there's just also this idea of being more transparent about what we're doing. Our studies are intended to help improve the lives of low-income college students in the postsecondary group. And we think that the data should just be available to any qualified researcher who can at least prove that they'll keep the data securely housed. And we want them to be able to look at it and analyze it and help us learn even more.
Leigh: That's great. Thank you. All right, Mike, you talked a bit about being able to gain substantive knowledge, which I really hear as helping to figure out how to make this information as useful as possible to people. And I know MDRC recently released its first publication on a specific topic using this massive database, a short brief titled “What Happens After the Program Ends?” So, tell us, what does happen after the program ends?
Mike: So this piece was inspired a bit by work that's been done in early childhood where a lot of researchers have looked at programs that maybe examine the effectiveness of some new prekindergarten program, a new curriculum, new way of approaching teaching and learning in that space. And they found that some of these programs seem to help students become ready for kindergarten and for elementary school.
But then as they've tracked them over time, there's been times where those programs see effects that are maintained, but then other times there appears to be some sort of fade out. And so, we were just interested in thinking about that same type of topic, but now in the postsecondary space.
And so, what we wanted to do was say, "Well, all right, we've looked at all these different programs and some of them last one semester, some of last one year, some of them last three years, but oftentimes we've tracked students’ progress after the program ends."
And we wanted to see what happens after the program ends, if there are effects early on during the program, after the program that they seem to grow. You can imagine a program, let's say it's a success course that teaches people study skills and time management, then maybe it helps you while you're taking the course, but then even afterwards, if you maintain those skills and keep using them, you can imagine the effects growing over time.
But you could also imagine that maybe effects are just maintained or on occasion maybe they fade out, maybe a group that doesn't receive some special program or policy, initially is a little bit behind, but then they just catch up and in the end, they're just kind of no difference.
And what we did was across all these different studies, we looked, what were the effects at the end of the program in terms of how many more credits students had accumulated, if they were part of some program. And then we just looked: Did that change over time? Did the control group tend to catch up? Did they maintain the gains that they'd made, or was it actually the case that the [effects] kind of grew even faster after the program ended?
And what we found, by being able to look across all these different studies, was a pretty consistent finding that most of the time effects appear to be maintained. For example, at the end of the ASAP program [CUNY’s Accelerated Study in Associate Programs] that we studied, after three years, people were around seven credits or eight credits ahead if they were offered this program compared to if they were not. And if you look another semester or another year later, the results are pretty much the same. They haven't gained any more credits compared to the control group, but the gap remains about the same. They maintained the effects that they achieved during the program.
And this was true consistently across interventions that were short-term, long-term, whether or not they were advising programs, or financial aid programs, or student success courses, or learning communities—pretty much across the board while the program was happening. Oftentimes there were positive effects, but then once the program was over, those effects were maintained. They didn't grow, but they also didn't shrink. There was no evidence of fade-out. So I think it's a pretty reassuring finding, unlike in that pre-K space where oftentimes they're finding programs that effects fade out.
Leigh: I love really thinking about what we've learned in other domains where MDRC works, like early childhood, and thinking about how to apply that to postsecondary education. What you're talking about feels like issues of real importance for college or system leaders thinking about how to support students. And it seems like good news so far in terms of there not being fade-out. What are some examples of other types of substantive questions that you hope to be able to answer using the database?
Mike: One of the most interesting things that we're hoping to do with this database is we're trying to understand what program features or components, things like financial supports or enhanced advising, or tutoring, or learning communities, which one of those components tend to be present in the programs that are having the largest effects, the programs that most effective, helping the students the most.
A challenge we face is that, in most of the evaluations that we've done, the interventions or programs that we've studied, they involve more than one component alone. They're not just advising, they're advising paired with financial support, or they're a success course and a learning community, or so some of them even have five or six components. And randomized controlled trials are fantastic at telling you what the overall average effect of such a package of services is, but they often aren't the best at trying to disentangle which components were the ones that matter the most.
We're trying to look across these 39 interventions and see: Are there certain components that were present in those interventions that had the larger effects? So maybe it turns out that most of the programs that had bigger effects, made people more likely to graduate, they had larger impacts on credit accumulation. They made people more likely to persist in college. Maybe they all had financial supports. And that would provide some suggestive evidence that that's a really important component to include in programs that are going to make a bigger difference for students.
So that's one really interesting question that we're trying to better understand: which of these components have the most evidence of their making a difference? And, as part of that, we're also going to try to confirm a hypothesis that we talk about a lot but hasn't really been kind of tested that rigorously in the past, which is: Are programs that are more comprehensive, meaning those that have more components, actually more effective?
And this is really important because I think we assume that they probably will be, but we want to both confirm that that assumption is true—and also try to get grasp of: how much more effective are they? Because more comprehensive programs we think probably are addressing more barriers to success, but they also cost more. So, when a college is trying to figure out where to start, we always want to tell them, "Do everything if you could, because all these things seem to be making a difference." We believe that financial support, advising, tutoring, all them are helping hopefully, but you’ve got to pick and choose sometimes if you can't afford to do them all at once.
And so, we want to get a sense of how much added impact is there as you start adding more and more components in there? And maybe it turns out you don't need to do all of them, but you actually get kind of the same bang for your buck if you were just doing two or three components instead of doing six of them. So that's going to be important question to look at, too.
A second thing we're looking into using this database—and this is with my colleagues, Austin Slaughter and Colin Hill—is about how to think about funding some of these different interventions. Colleges often think mostly about the cost of implementing a program. And a lot of the programs that are in this big data, we've documented what the cost is to implement them. But it's also important to consider the revenue that they might generate. For example, if a program has positive effects on student outcomes like retention, then the college will get more money from tuition revenue. If more students come back the next semester, they get tuition revenue. And similarly, a lot of states provide performance funding. So, if students earn more credits than they would have, or they graduated at a higher rate, or they make their way through developmental education at a higher rate, that actually triggers more state funding for a college.
We're trying to build a tool that colleges can use that helps them consider for each intervention in this database, both the costs of implementing the intervention, but also the revenue that it might generate to them that would offset some of those costs. So that when they're making these decisions, they're not just worried about the cost and how they can fund it, but they're also thinking about the potential offset of some of those costs from the revenue that they generate.
Leigh: All right. So it feels like that tool that you're talking about could be incredibly useful for college or system leaders, [who are] really trying to think about how to allocate scarce resources. But you mentioned that it needs to take into account state policies about funding formulas, but it feels like those policies likely change over time. Will this tool be updated to actually document those change and policies?
Mike: Version 1.0 of the tool will involve around 35 states, and we've built into the tool their state funding formulas. The really cool thing for people that want to use this is eventually you'll say, "I'm in Texas. And I want to know if I implemented a program that was actually implemented in Ohio, what the implications will be in terms of the costs." And we've done regional price adjustments. So, we'll give you the price in your actual specific location. But then also the revenue; you want to make sure it's grabbing your college's tuition. It's going to do that because, in the back end, it has each college tuition rates. And then also in the back end, it has each of these 35 states’ state funding formulas.
So built in will be, in Texas, we'll give you X additional dollars for credits and Y additional dollars for graduation. So we will have that. Our hope is that we'll continue to update it over time. We don't have funding for that as of today. But listeners who are funders, you might think about that as being a great thing to support us with as we move down the road.
One thing we're actually trying to look at as we developed a tool is: the initial round of looking at these state funding formulas came from a prior year. And then we're going to update it before we actually officially launch with the current year's funding formulas. So, as we're doing that, we're trying to get a sense of how much do these things really change from year to year? Our suspicion is that, probably, you can go a couple years without major consequential changes, but maybe every five years it would really be worth updating this.
But another thing we're doing that's built into the tool is that users can override our inputs. So, we'll be clear what year we've developed this for, and, if your state's funding formula has changed, it will be possible to override it.
Now, part of the nice thing about the tool as it's designed now is you don't have to go find that information out, because it takes a lot of work actually to decipher state's funding formulas and understand how much reward there really is for each of these outcomes. We've done all the hard work for you. So, that's kind of the nice thing. But if we don't ever find funding to update all this in 2025, then there is the option for people to do it themselves and still take advantage of all the other features of the tool.
Leigh: That's very cool. I'm really excited for that to be something that's going to be available to people, because it sounds incredibly useful. All right. I know that a lot of your research is about trying to figure out how to best support community college students and their pursuit of academic success, and helping colleges figure out how to use the information to inform their decision-making. But I know that you also conduct lots of methodological research to improve how we do evaluation. Can THE-RCT help improve how we do our work, how we do evaluations?
Mike: Yeah. So, you're right. A second strand of the work that I do, and also that MDRC does, is to try to help improve how we design implement and conduct evaluations. And my specialty is in the space of randomized controlled trials. And one thing that was sort of missing in that space—in the postsecondary world at least—was information that's needed for the planning of randomized controlled trials.
When you're beginning the planning of a trial, one of the most important things you have to figure out is: how many colleges do I need to be involved? How many students need to be involved? So that at the end I can be pretty confident in my findings, so that I will know that my estimate of the effectiveness of the intervention is precise enough that I can feel really good about knowing: did this thing make a difference? How big of a difference was it?
And so,, there's this thing called design parameters. They're basically just the numbers you plug into a formula that help figure out: if I had this size sample, how small of an effect would I be able to detect? And we call these calculations, the minimum detectable effect calculations. Prior to the work that we're doing right now, there was very limited information about what numbers to plug into those formulas. So you have to know how well baseline information about people—their race, their gender, their age—how well do these predict the outcomes? If they're very predictive, you actually can use a smaller sample size and still detect effects of the same size. So that's one piece of information you need to know.
You also need to know how much the outcome varies. For example, if your main outcome in a study was to look at “how much did intervention X improve credit accumulation through a year?”—you actually need to know how much does credit accumulation at the end of the year vary across students that are in the study.
I don't know what people did prior to what we're producing—when they're trying to figure these numbers out, that you need to plug in to figure out the sample size. I'm not sure if they're just sort of guessing; maybe they're using information from K-12 where there's been a ton of work on this kind of stuff. But in postsecondary, as far as I know, there's been nothing done.
We are working on trying to provide to other people information from all of these different studies about what those design parameter values were. The numbers they would need to plug into their calculations to figure out the sample size. Our hope is that this will lead to people being able to do studies that are more informed. When they're trying to do the planning to make sure that their sample sizes are either adequately large, that they can detect effects that are of a meaningful size, but also maybe not too large, they're not just throwing money away, right?
These trials are very expensive. And if you plug in the wrong numbers to these formulas, you might end up thinking you need a much bigger sample than is necessary. Usually, it's probably the other direction that people think they can get away with a smaller sample size than they really need, but we're trying to help provide the information that will enable researchers to do that in the future.
So that's one sort of methodological [advance] that we're working on taking advantage of this very same database. Another one we're working on relates both to the planning of studies and then also how you interpret the results at the end. This one is to look at empirical benchmarks for understanding what kind of effects we have seen in the past. So, the idea here is: let's say that you're going to plan a study in this case. And you know that if you have a sample size of 500 people that you can detect an effect of two credits earned at the end of a year. You might want to think to yourself, well, has anyone ever seen that size effect before in a study? And if the answer is no, then probably you need a bigger sample size because you'd have to see the biggest effect anyone's ever seen. And that would be kind of worrisome, I think.
So, it might be helpful to see across these 30 different studies we've done, what is the distribution of effects on credits earned through a year? What does it look like? Maybe it's ranged from zero all the way up to two or three credits. You just might want to know that to help figure out how well-positioned am I to detect the size effects that I can detect when I'm planning a study.
But then the same thing applies on the flip, and at the end of the study, you get your results in. And one interesting way to potentially interpret the results is to compare them to what we've seen for past interventions. So, again, you do your study, maybe it's a new financial aid reform. Someone wants to know if I increase Pell grants by a thousand dollars, how big of an impact would that have on students’ credit accumulation through a year? You get your estimate at the end; let's say it increases credit accumulation by a credit. Well, how do you think about that? I mean, some ways you can think about that would just be, well, most classes are worth three credits or four credits—so, maybe that means that around a third or 25 percent of the sample passed one extra class.
But another useful way to position that finding would be to say, "Well, where does that fall among all different interventions that people will have tested in the postsecondary space? Is it similar to some of the other interventions? Is it much bigger? Is it much smaller?" And so that's what we're hoping to provide people with information, some context for interpreting findings, relative to what we've seen in other interventions.
And in doing this, we'll show people, both what the whole distribution looks like, but then also each individual intervention. If you want to say, "Well, my intervention was in the financial support realm," you might actually care most about looking at other financial support interventions and saying, "How do the effects of this one compare to those?" So we'll make all of this information available, kind of like looking at the broad distribution, just all these interventions, what size effects they've had, you could kind of just say, "Well, I just want to look at the intervention that seemed most in the same field as mine or space as mine, or intervention type as mine.”
Leigh: That is very cool. That's really exciting for the research field. How can other researchers gain access to THE-RCT’s restricted access file to explore other questions of interest?
Mike: To access this database, you can go to the Inter-university Consortium for Political and Social Research, or ICPSR, which is hosted at the University of Michigan. The website is something like icpsr.umich.edu. But you can just go Google it if you're not sure where that is.
And they have a search bar and you can just type in THE-RCT or MDRC’s THE-RCT, if you want to get access to this information. Importantly, there's a lot of information that you can literally just download right now. You can just go and all the documentation for the data [is there]. There's an RCT database, which has each study as a row. And then just a lot of information. What was the sample size? How many program group members? How many control group members? What was a description of the intervention under study? What was a brief summary of the implementation findings? The cost findings, all those types of things are all in there, as well as links to reports—if you want to get into real detail for each individual study. All that information, as well as a user's guide, you can download immediately.
To access the individual-level data, there are some hoops you have to jump through, and they're important hoops, because we want to ensure the security of this data. But you can find all that information on ICPSR's website. It's stuff like demonstrating that you have a principal investigator for your project, that you have some research questions, but most importantly, it's providing information about how you're going to ensure that the data is securely housed and promising that you're not going to try to re-identify students that are in the files.
If you have questions, you should feel free to reach out to ICPSR—definitely if it's about downloading the data. But if it's more about understanding what's in it, you're welcome to reach out to me at michael.weiss@mdrc.org or my colleague John Diamond, who is integral in the creation of the database. He’s a real data guru who put up everything together. He's John J-O-H-N.diamond@mdrc.org. And he also can help out with information. Because the database I think is very useful, but there's a lot in there. So, there's a lot of difficult decisions we had to make, and we're happy to sort of talk through what we did and how we did it and why we did it.
I'll also add a little pitch that anyone who's listening who's working on their own randomized controlled trial. Currently, this database only includes MDRC trials, but that is not because that's how we want it to be. We'd love it to be including other people's RCTs too, so it's even easier for everyone in the field to do these kinds of syntheses. We already are working with RAND to get their Single Stop evaluation to be included in this. That's still a few years down the line because they're only just beginning it, but we're really happy that they're partnering with us on that. But we'd love other people to include their studies in this, too. So please reach out if you're interested in getting your data included in this, too.
I know anyone who's worked with Arnold Ventures or IES, it's now pretty much a requirement to make your data into a restricted access file. So, we would love to partner with people when they're doing that so that it's not just out there in the ether, but actually in a single repository that makes it really easy for people not just to look at any one study, but look across all these studies.
Leigh: All right. Mike, thank you so much for joining me today. This has been really interesting, and I think the work that you're doing is going to be really useful for the field and for other researchers. And I hope that people will make use of this database.
Mike: Thanks for having me, Leigh. It was fun.
Leigh: To learn more about MDRC and THE-RCT project, visit mdrc.org. Did you enjoy this episode? Subscribe to the Evidence First podcast for more.
About Evidence First
Policymakers talk about solutions, but which ones really work? MDRC’s Evidence First podcast features experts—program administrators, policymakers, and researchers—talking about the best evidence available on education and social programs that serve people with low incomes.
About Leigh Parise
Evidence First host Leigh Parise plays a lead role in MDRC’s education-focused program-development efforts and conducts mixed-methods education research. More